Labeling Training Data: The Key to Success in AI Development
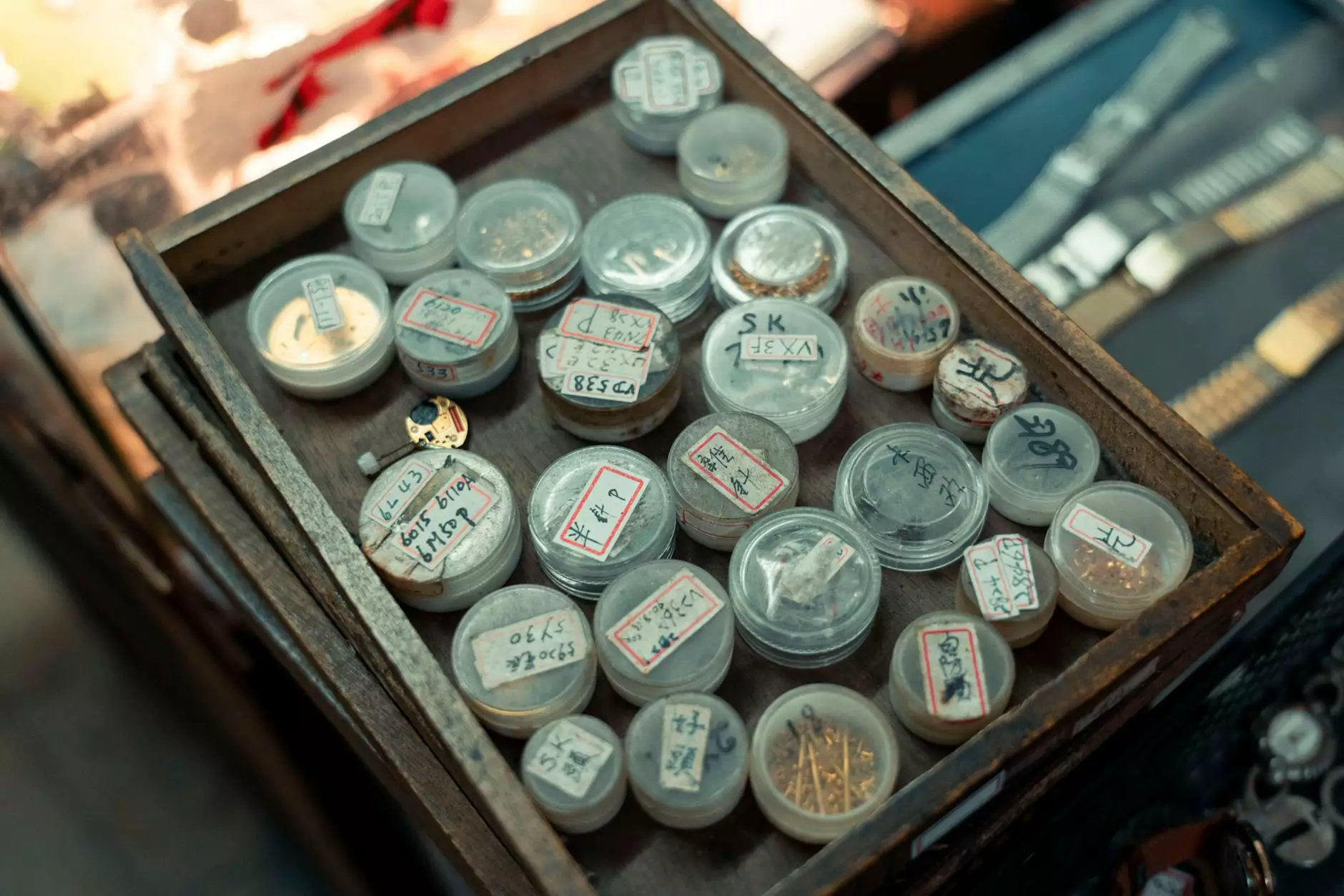
In the rapidly evolving world of artificial intelligence, the importance of labeling training data cannot be overstated. As businesses look to harness the power of AI, the groundwork for successful models lies in the quality and accuracy of the training data. At Keylabs.ai, we specialize in providing cutting-edge Data Annotation Tools and a comprehensive Data Annotation Platform that streamline the labeling process. This article delves into the intricacies of labeling training data and its significance in the development of high-performance AI systems.
Understanding the Role of Training Data in AI
AI models, particularly those based on machine learning, rely heavily on training data. This data serves as the foundation upon which models learn and make predictions. Without properly labeled training data, even the most sophisticated algorithms can falter. Here are several key points to understand:
- Quality Data is Crucial: The saying "garbage in, garbage out" rings true in AI. Poorly labeled data can lead to incorrect predictions, while well-labeled data helps create robust and reliable models.
- Data Types Vary: Data comes in numerous forms including images, text, audio, and video. Each type requires a unique approach to labeling that fits the specific needs of the model being developed.
- Volume Matters: For complex AI systems, vast amounts of data are necessary. The more data, the better the model can generalize from the training it receives.
The Process of Labeling Training Data
Labeling training data is a meticulous process that involves multiple steps. Understanding these steps can enhance how businesses approach data annotation:
1. Collecting Raw Data
The first step in labeling training data is collecting raw data. This involves amassing data from various sources relevant to the task at hand. For instance, if the goal is to create an image recognition model, the data collected should include a diverse range of images encapsulating the objects of interest.
2. Choosing the Right Annotation Tool
Once the raw data is collected, the next step is to select an appropriate Data Annotation Tool. Here’s what to consider:
- User-friendly Interface: The tool should be easy to navigate.
- Type of Data Supported: Ensure it can handle the specific type of data you are working with.
- Integration Capabilities: The tool should seamlessly integrate with existing data workflows.
3. Annotation Guidelines
Clear and comprehensive guidelines are essential when labeling training data to ensure consistency across the dataset. This may include:
- Label Definitions: Detailed descriptions of what each label means.
- Examples: Providing examples for annotators helps to clarify any ambiguities.
4. Human Annotation
The actual labeling is typically performed by human annotators who apply the defined labels to the collected data. This process is crucial for achieving high-quality training data. With the aid of advanced Data Annotation Platforms, tasks can be distributed among multiple annotators to enhance efficiency.
5. Quality Assurance
To ensure the accuracy of the labels, a quality assurance process must be implemented. This process can involve reviewing a sample of labeled data, inviting feedback from annotators, and refining guidelines or tools based on observations. Regular audits can also help maintain high-quality standards.
The Benefits of Effective Data Labeling
The process of labeling training data is not just a hurdle to overcome in AI development; it offers numerous benefits:
- Improved Model Accuracy: High-quality labeled data results in models that make more precise predictions.
- Faster Time to Market: Efficient data annotation processes can accelerate development timelines.
- Scalability: Advanced tools and platforms allow projects to scale as data needs grow.
Common Challenges in Labeling Training Data
While labeling training data is essential, it is not without its challenges:
- Subjectivity: Different annotators may interpret guidelines differently, leading to inconsistencies.
- Time-Consuming: The process of labeling large datasets can be labor-intensive and slow.
- High Costs: Depending on the complexity, recruiting and training annotators can be expensive.
Leveraging Technology for Efficient Data Annotation
To address the challenges in labeling training data, many businesses are turning to technology. Utilizing a state-of-the-art Data Annotation Platform can significantly mitigate these obstacles:
1. Automated Labeling
Automation can improve efficiency in labeling. For instance, machine learning models can assist in initial labeling, which humans can then refine. This hybrid approach speeds up the process.
2. Collaborative Workflows
Modern platforms facilitate collaborative workflows, allowing teams to work on data simultaneously. This collaboration enhances productivity and ensures that projects at Keylabs.ai can adapt swiftly to changing business needs.
3. Continuous Learning
Machine learning systems can continue to learn from the data they process. The insights gained from data can be fed back into the model to improve its performance continuously.
Conclusion: The Future of Labeling Training Data
In conclusion, the significance of labeling training data in the AI landscape is profound. As more businesses embrace AI technologies, the demand for quality data annotation will continue to grow. By leveraging advanced Data Annotation Tools and a comprehensive Data Annotation Platform, organizations can ensure that their AI models are built on a solid foundation of quality training data. Investing in proper data labeling not only improves model performance but also enhances the overall success of AI initiatives.
Embrace the future of AI with confidence by prioritizing effective data labeling strategies. At Keylabs.ai, we are committed to providing the best data annotation services to help you achieve your AI goals.