Understanding Email Spam Filter Machine Learning
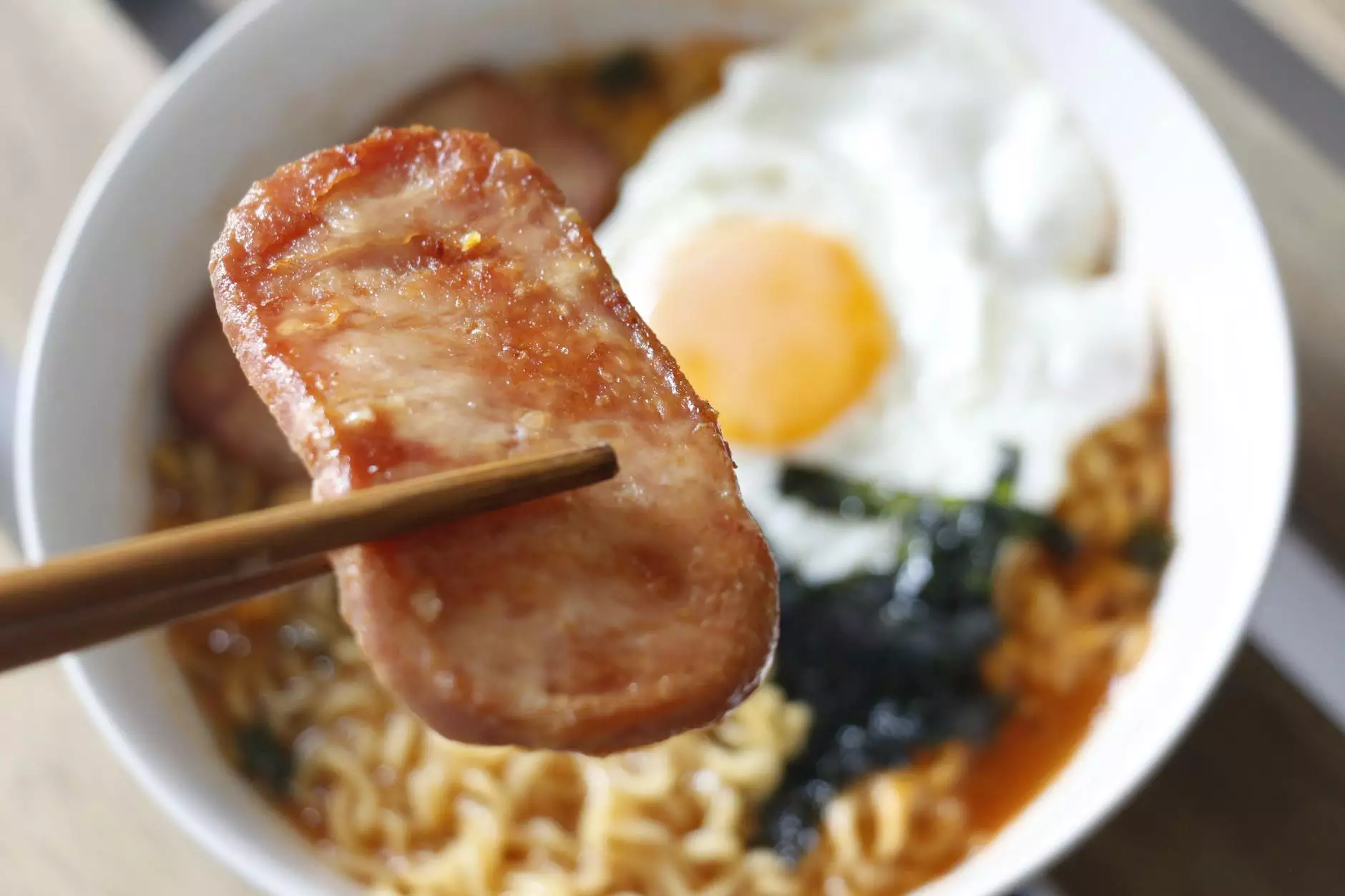
Email is a critical communication tool in today’s business landscape, but it also comes with challenges, particularly the ever-present threat of spam. For businesses to thrive, they need robust solutions to protect their inboxes from unwanted messages. This is where email spam filter machine learning comes into play. It’s a game-changing methodology that not only enhances the security of email communications but also optimizes user experience. In this article, we will delve deep into the intricacies of machine learning spam filtering, its benefits, and how it is transforming the world of email security.
The Rise of Spam: A Persistent Challenge for Businesses
Spam emails have been a nuisance since the early days of the internet. From unsolicited advertisements to phishing attempts, spam not only clutters email inboxes but also poses serious security risks. Businesses are particularly vulnerable as cybercriminals exploit vulnerabilities to infiltrate systems and steal sensitive information.
- Increased Cybersecurity Threats: With the rise of sophisticated cyber attacks, businesses face heightened risks from spam that can deploy malware.
- Loss of Productivity: Employees spending valuable time sorting through spam can lead to decreased productivity.
- Reputational Damage: Accidental engagement with spam can lead to breaches, damaging a company's reputation.
How Machine Learning Enhances Email Spam Filtering
Traditional spam filters often relied on simple keyword matching and blacklists. These methods were effective in the early days but have increasingly been outmatched by the evolving nature of spam. Machine learning brings a more sophisticated approach by leveraging algorithms that learn from data patterns.
What is Machine Learning?
Machine Learning (ML) is a subset of artificial intelligence that enables systems to learn from data, identify patterns, and improve their performance over time without explicit programming. In the context of email spam filtering, machine learning algorithms analyze vast amounts of email data to differentiate between legitimate messages and spam.
Key Mechanisms of Machine Learning in Spam Filtering
Machine learning algorithms can utilize various methodologies to enhance spam filtering:
- Supervised Learning: In this technique, models are trained on labeled datasets where emails are classified as either spam or legitimate. The algorithm learns to identify features common to spam messages.
- Unsupervised Learning: Unlike supervised learning, this approach does not rely on labeled data. Instead, it groups similar emails together, allowing for the discovery of unknown spam types.
- Reinforcement Learning: This adaptive learning mechanism allows the spam filter to improve over time based on feedback from user interactions, effectively decreasing false positives and negatives.
Benefits of Using Machine Learning for Spam Filtering
There are numerous advantages to integrating email spam filter machine learning into your email security protocols:
1. Improved Accuracy
Machine learning algorithms can achieve higher accuracy rates than traditional filters by identifying sophisticated spam patterns that may be overlooked. This minimizes false positives where legitimate emails are marked as spam.
2. Adaptability
Spam tactics are continually evolving, requiring an adaptive solution. Machine learning models adjust in real time to new threats and spam trends, ensuring that businesses are always protected against the latest spam technologies.
3. Automation and Efficiency
By automating the spam detection process, machine learning eliminates the need for manual filtering. This significantly reduces the workload on IT departments, allowing them to focus on other critical tasks.
4. Enhanced User Experience
A more rigorous spam detection process means employees can focus on important emails rather than wasting time sifting through spam. This leads to improved overall productivity within the organization.
Implementing Machine Learning Spam Filters
Implementing a machine learning-based spam filter is essential for businesses wanting to enhance their email security. Here are steps companies should consider when integrating this technology:
1. Assess Your Current Email Environment
Before implementation, conduct a thorough analysis of your current email systems and existing spam filtering solutions. Understanding your baseline will help tailor the machine learning solution to your specific needs.
2. Choose the Right Machine Learning Algorithm
Different machine learning algorithms can be employed for spam filtering. Choose one that best fits the volume and type of emails your organization handles. Popular algorithms include Naive Bayes, Support Vector Machines, and neural networks.
3. Collect and Prepare Data
Gather historical email data to train the machine learning model. This data should include both spam and legitimate emails to help the algorithm learn the distinguishing features.
4. Train the Model
With your data prepared, train your selected model. This involves fine-tuning the algorithm’s parameters and validating its performance using test data. Evaluate the model's accuracy and efficiency in identifying spam.
5. Monitor Performance
Once deployed, continuously monitor the performance of the spam filter. Regularly update the training data to adapt to new spam techniques and enhance the model's accuracy.
Future Trends in Email Spam Filtering and Machine Learning
As technology evolves, so do the solutions for combating email spam. Here are a few trends we can expect in the realm of email spam filter machine learning:
- Integration with Threat Intelligence: Spam filters will leverage real-time threat intelligence to proactively address emerging spam threats.
- Natural Language Processing (NLP): Enhancements in NLP will allow machine learning models to better understand the context and semantics of emails, improving spam detection accuracy.
- AI-Driven User Education: Future spam filters may integrate user education, guiding employees on how to spot potential threats and securely handle suspicious emails.
Conclusion
In a digital world where spam continues to pose significant challenges to businesses, the integration of email spam filter machine learning is not just an advantage but a necessity. This innovative solution not only enhances email security but also fosters a more productive workplace. As we move forward, embracing these technologies will be essential for companies wanting to safeguard their communications and maintain robust cybersecurity postures. The investment in a machine learning-based spam filter is an investment in the future of secure and efficient email communication.